Connections
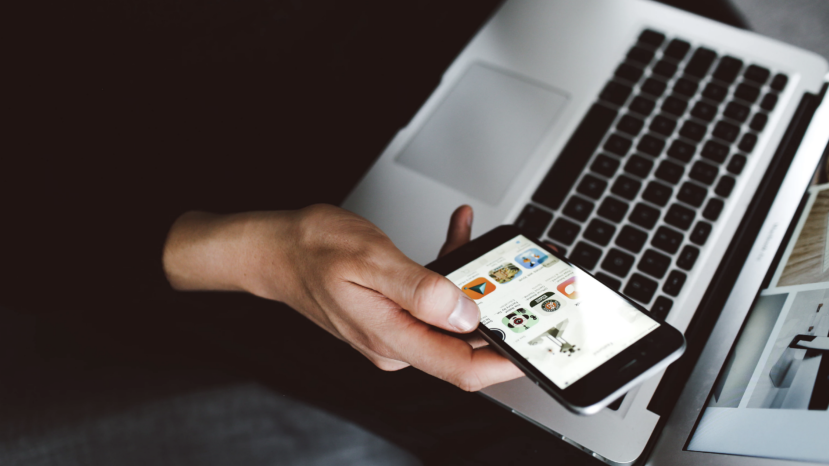
M&E Journal: The Disruptive Technologies: Connecting and Converting Targeted Advertising
Story Highlights
By Lucretia Williams, Global Marketing Director, Luxoft –
The M&E industry has always valued the creative process, from the story idea and narrative to distribution. In the past, consumer consumption was limited to the top three networks on the family television set (ABC, CBS and NBC) and the local movie theater, creating a market for a one-to-many advertising approach. Today, content is readily available anytime, anywhere, on any size screen, causing a shift to more data-driven decisions.
How can big data help us find films we haven’t even heard of, but are likely to love?
Although recommendation systems have been around for a decade, artificial intelligence, machine learning and access to mountains of data have breathed new life into this antiquated methodology. The old systems created applicable guesses — sometimes unexpected but in most cases fairly accurate — for movies, music or products the end user would enjoy. Even so, it often left consumers feeling overwhelmed by massive amounts of content choices.
Researchers have since discovered that customers complete their purchases when visiting sites to locate a specific item. What is interesting, however, is that purchases were typically made when re-visiting the site to buy something unrelated to what led them there in the first place. These observations, combined with a growing “must guide” user-experience in stores and online, led to the active development of systems that predict shopping behavior and provide relevant purchase suggestions. We now call them recommender systems.
Here is where big data comes into play. It identifies patterns and connections that can’t generally be explained on their own. And as data sets become deeper and more multidimensional, companies will discover correlations and be able to provide recommendations with more precision, while simultaneously implementing virtual biometric technologies. We are still on the cusp of understanding how to apply advanced logic, data-driven algorithms, especially with the implementation of biometric measures upon the horizon.
How do recommendation systems work?
One of the first strategies is collaborative filtering — creating machine-driven predictions of a user’s interests by collecting the preferences of multiple users. Collaborative filtering applications typically involve huge data sets. As in all big data analytics, the predictions from recommendation systems are specific to a user, while collecting information from other anonymous users. These systems begin with finding users who share the same rating patterns with a targeted user, and then assessing the ratings from like-minded users to calculate a prediction for the targeted user.
Another common methodology for recommendation systems is content-based filtering, which leverages algorithms that recommend items similar to those the user has liked in the past. This encompasses a weakness, however, since the algorithm rule can’t show new and unknown product areas the user may additionally like. As a result, hybrid filtering systems are typically used for overall accuracy.
AI and algorithms: The investment
Netflix was an early adopter of experiential recommendation applications. Netflix’s technology and development budget has increased slightly faster than its overall revenue. Last year, it increased by 38 percent to $651 million (about 10 percent of its revenue), however, not all of this spend goes into its recommendation engine.
According to Netflix’s 10-K, technology and development team expenses include “testing, maintaining and modifying our user interface, our recommendation, merchandising and streaming delivery technology and infrastructure.” All of these factors have contributed to its AI algorithms, saving Netflix $1 billion each year, not to mention the effects on its churn rate with a meaningful improvement to make a retention difference of even 0.1 percent,” reported by VP of Product Innovation Carlos Uribe-Gomez and Chief Product Officer Neil Hunt.
Next level: the power of algorithms
The power of algorithms boosts performance levels, manufacturing real business value as the cloud improves the speed and capacity to analyze mountains of data. New content is consistently being created by M&E broadcasters and self-publishers, rapidly expanding the catalog of new and old content available to consumers.
AI and machine learning make it possible to work with large catalogs of data, enabling viewers to find whatever they desire – even when they are not quite sure what that might be. Their success can be attributed to the ability of algorithms to boost subscriptions and consumer engagement despite the increase in streaming options.
The horizon of blockchain
Traditional advertising has operated on a one-to-many approach based on reach, limiting the ability to target an ideal customer with precision. As customer IP TV consumption increases, advertising will become more addressable, and a one-to-one approach is an excellent framework for blockchain. M&E companies are starting to implement blockchain into their digital stack. Marcien Jenckes, President of Advertising at Comcast Cable, said at CES: “Comcast is also working on a way to use blockchain techniques in the connected home in a way that safely provides customers a way to grant and revoke access to their IoT devices and smart home applications.”
As blockchain continues to evolve recommender systems, there will be even greater personalized user recommendations. Just think for a moment of all the binge-worthy shows and viral videos you discovered while not even searching for them! With higher quality data and new emerging technologies available, this will allow for better targeting of addressable ads and viewer content to maximize results, which improve customer engagement, reduce churn and increase revenue.
M&E technology leader
Luxoft’s approach is rooted in governing the ever-increasing complexity of industry trends, with customer data, personalization, customization and automation as drivers behind chatbot, blockchain, AI, BPM and machine learning. Our managed delivery model is underpinned by a highly-educated workforce, allowing continuous innovation upwards on the technology stack to meet evolving digital challenges.
—-
Click here to translate this article
Click here to download the complete .PDF version of this article
Click here to download the entire Spring/Summer 2018 M&E Journal