Connections
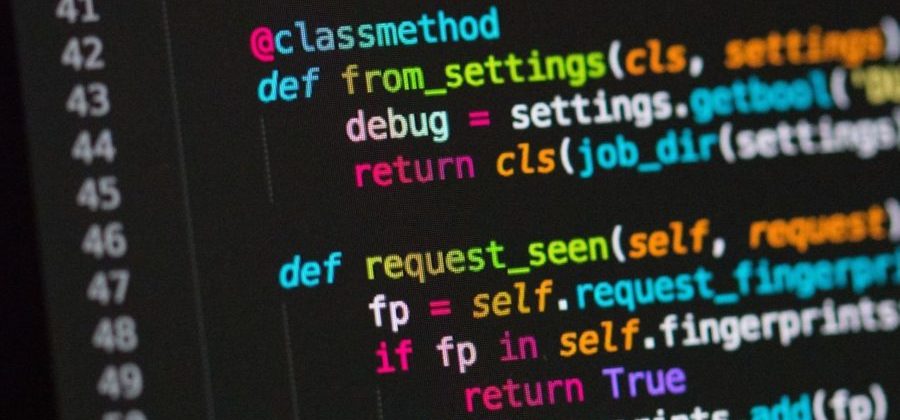
M&E Journal: Using Machine Learning to Transform Creative Talent Management
Story Highlights
There’s an old saying in business: Your biggest assets (people) leave in the elevator every night.
That’s doubly true in the creative talent management business, where your products (actors, directors, writers, sports stars and musicians) leave, albeit via Tesla, Uber, Toyota Prius or a Trek Domane SL 7 bike. Talent is defined by a rich and disparate set of data, both structured and unstructured (such as audience data derived from social media streams).
Data consists of everything from every job the client has ever had, to how viewers across the globe are “consuming” them, to industry knowledge about future projects. It’s no longer just a small analytics group that needs access to this data. It’s now also the talent manager who must mine these data resources, bridging the gaps and making the connections that comprise each story.
The plot thickens: Dirty, duplicate data
Traditionally, a lot of that data has resided in the heads, smartphones and spreadsheets of individual talent managers and their teams, who collect, connect and use it to discover and get the best roles for everyone involved. Today, as in every other industry, data — and the speed and predictability with which talent managers can act on it — is a competitive advantage.
But the traditional process no longer works. In a world increasingly dominated by data-driven digital media superpowers like Netflix and Amazon, talent management must follow suit. Technologies like the cloud, data warehouses and lakes, and easy-to-use business analytics are becoming table stakes. The catch is that it all hinges on analytics-ready data for talent managers to use.
But it’s elusive. Problems include dirty and duplicate data, which obfuscates a clear, current picture of a talent or a brand. “Data drift” happens when critical changes and updates on the front lines never make it back to the source. Data experts and IT people spend an inordinate amount of time “unpacking” clusters of disparate data sources that have drifted or become dirty or duplicated. Given the increasing amount and diversity of data, it’s usually impossible to ever catch up when using manual or marginally automated data-engineering methods.
Meanwhile, starved of clean, accurate data, those stories in the heads of talent managers go into perpetual rewrites — or on hiatus.
Applying machine learning
A leading creative agency representing talent, brands and enterprises found a better way: Use machine learning (ML) to do the heavy “data-lift,” then turn over the results to their own internal data experts to make it perfect.
The agency’s script called for a built-to-last data factory designed to virtually centralize the agency’s data assets and speed up analytic velocity. The first project: “people mastering.” Apply ML to existing data to create a novel 360-degree-view of talent that would equip talent managers with data-backed insights to make decisions. The agency built data pipelines from sources to a factory warehouse, with Power BI analytics dashboards for talent managers on the other end.
A single, unified view (master entity) defined each kind of person (whether actor or director, musician or tour, team or athlete), capturing and presenting data from multiple sources such that media, entertainment and sports companies could maximize revenue in a variety of ways. This mastering process would later be applied to media titles and other business entities.
The goal was to make time-to-insights as fluid as possible. The missing link: ensuring that master entities were always current and up to date, even though the underlying data was ever-changing. With previous methods of entity-mastering maxed out, the agency invested in novel, human-guided machine learning technology. Once trained, machine learning models of the entities were able to handle up to 90 percent of updates solo, efficiently involving people familiar with the data to resolve the remainder.
A (close-to) Hollywood ending
This approach has dramatically improved the fidelity of the data, even as it grows in volume and variety. Freed from much of the burden of data updating, data experts can now focus on creating more and better analytics and improved dashboards for talent managers.
Managers can get fast, trustworthy answers to questions such as “Where has my musician not played in the last six months?” and, more importantly, “Where should they play on their next tour?”
With the new system, managers are better equipped with data-backed insights to make decisions. Their value — to the clients they represent and the media enterprises they serve — has increased. Both sides now have the unbiased data they need to make a fair negotiation. The best talent managers instinctively do this. With clean, analytics-ready data on tap, the agency can now scale that behavior out and up.
Finding new clients is easier, with the ability to get insights like “the fan base for actor A is similar to the fan base for actor B.” The agency has in fact built an auto-recommender for such questions, suggesting next-best action.
As needs change in response to media clients’ demands, it’s easier for data experts to create new analytics. For example: to respond to a studio directive about diversity in films, the agency could get fast answers to questions like “For a given film genre, how does cast diversity influence the type of audience we can attract?”
Further, the agency can ensure lasting success by being able to easily onboard new data sources for each entity as they become available, with the machine doing most of the updating work.
With the help of a machine, the human superpowers of a creative-management business are put to best use.
* By Matt Holzapfel, Solutions Lead, Tamr
———————-
Click here to translate this article
Click here to download the complete .PDF version of this article
Click here to download the entire Spring/Summer 2020 M&E Journal